Part 2: How AI is rewriting digital delivery
The consulting model we’ve relied on for the past two decades is shifting. Not all at once. Not everywhere. But the signals are clear. We’re starting to deliver differently. Or at least—we should be.
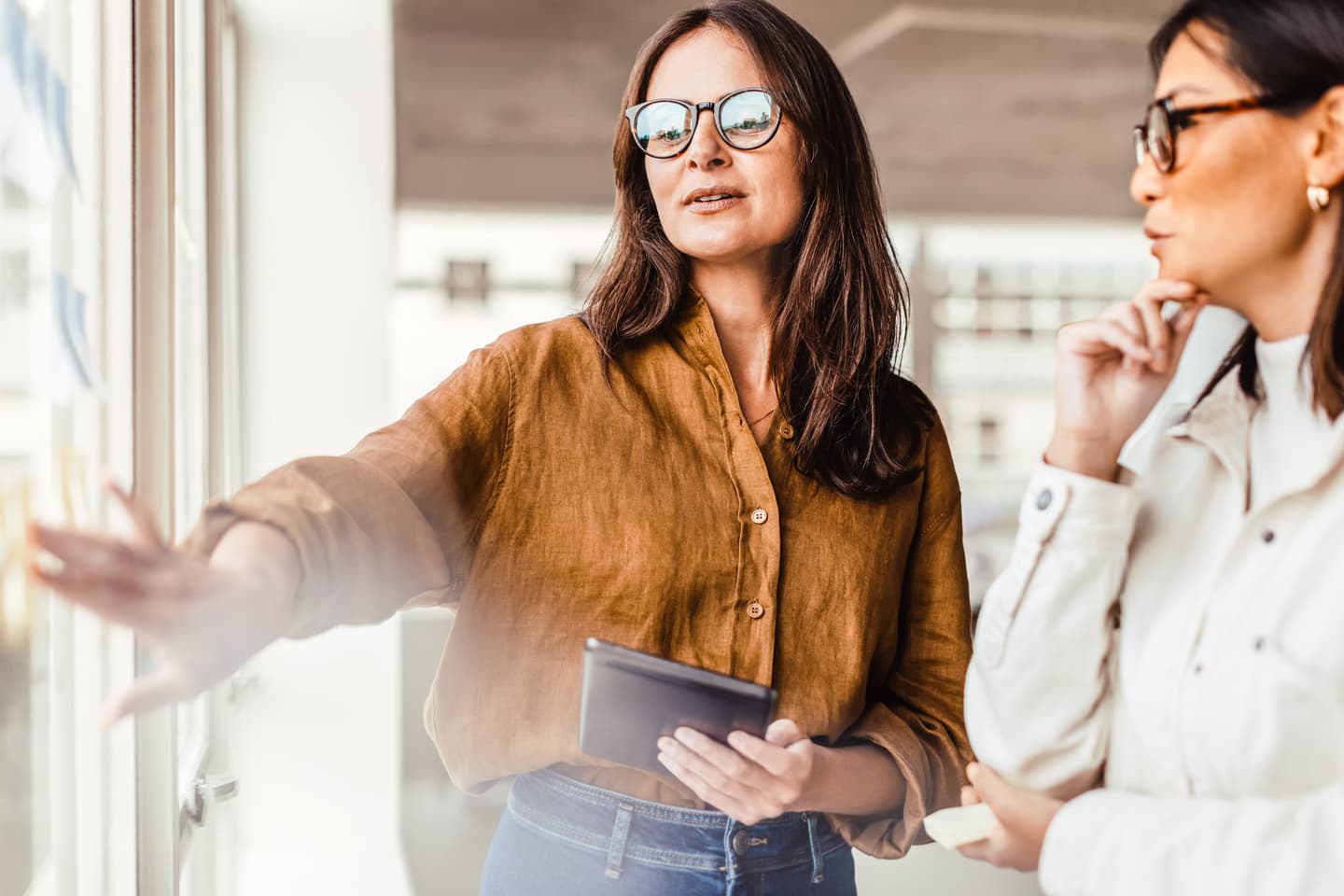
As a digital transformation consultancy, we’ve spent years helping clients move faster, modernise systems, and embrace new technologies. But now, the pressure to transform has come for us too.
Clients want more impact. They want it faster. And AI tools are giving teams a way to rethink how work gets done, what needs human input, and what doesn’t.
This isn’t about replacing people. It’s about building a new kind of consulting delivery—leaner, sharper, AI-augmented, and built for speed.
We’re not there yet. But the direction is set.
From scale to precision
Traditional digital consulting has often relied on scale—larger teams, longer projects, more hours. That made sense for complex transformation programmes with uncertain requirements and messy systems. It still does, in some cases.
But GenAI has started to rewire the economics of delivery. You don’t need ten people to do what three can now do with the right tools. You don’t need weeks of preparation to reach a first insight. You can collapse timelines—not by cutting corners, but by augmenting teams.
AI-native consultancies focus less on headcount and more on output. They build leaner delivery teams, structured around automation, orchestration, and faster feedback. Not because it’s cheaper—but because it’s better.
What can actually be automated (and what can’t)
We need to be clear with ourselves—and with clients—about what GenAI can actually do well today. It’s already strong at:
- Summarising transcripts, documents, or threads
- Drafting emails, slides, strategy narratives
- Generating and refactoring code, scaffolding UI components
- Generating design variants, alt flows, and microcopy
- Synthesising research and identifying patterns
But it’s not reliable for:
- Unsupervised analysis of novel or ambiguous problems
- Making final decisions in ethically sensitive areas
- Facilitating stakeholder alignment or negotiation
- Integrating conflicting data into a coherent recommendation
- Understanding organisational nuance or context
In short: it’s excellent at tactical work. It’s bad at context, nuance, and trust.
That’s why, in the short term, GenAI is mostly impacting junior-level delivery tasks—the kind handled by analysts, designers, and developers. Over time, though, agents will climb the stack.
Where Agentic workflows fit in
Most firms are still using LLMs to complete isolated tasks. But the next shift is agentic workflows—systems that perform multi-step sequences with minimal human guidance.
You might soon have agent workflows that handle things like:
- Taking a brief, querying trusted information sources, summarising insights, and drafting a strategy section for a deck
- Testing a prototype in Figma, flagging UX bugs, logging them in Jira, and tagging the right team
- Tracking project status across Notion, Slack, and GitHub—and nudging people before blockers escalate
In practice, these workflows may be powered by collections of agents working together. But from the team’s perspective, they’ll feel like a single, intelligent assistant—handling more than just a task. Driving the work forward.
We’re not quite there at scale. But with platforms like LangChain, AutoGen, CrewAI, and OpenDevin, we’re getting close.
And this matters, because ways of working are now the battleground for digital consultancies. Not proprietary frameworks. Not headcount. Not day rates.
The firms that figure out how to blend people, tools, and agents into seamless delivery will win.
Synthesised data: Still the foundation
Digital consultancies have long used data to support decision-making. But AI-native delivery changes the scale and speed at which that data is surfaced, processed, and acted on.
We’re now able to:
- Combine internal knowledge, client material, and external sources instantly
- Extract patterns and tensions across qualitative inputs in minutes
- Generate working assumptions and frameworks before the first workshop
Use synthetic inputs to simulate customer behaviour or feedback in early sprints These aren’t shortcuts. They’re capability shifts—from collection to synthesis, from backlog to momentum.
From playbooks to toolchains
Where traditional consultancies codify methods into playbooks, AI-native consultancies build toolchains. That means:
- Developing internal agents that automate routine documentation, story generation, and research
- Using structured prompt libraries that reflect brand voice, industry language, and client nuance
- Linking tools (Figma, Jira, Slack, GitHub) with orchestrators to automate check-ins, summaries, and escalation
- Owning the full flow—from prompt to outcome, with audit trails and human checkpoints built in
This isn’t theoretical. This is operational infrastructure.
And over time, firms will start to own more IP—not just knowledge, but software. The AI-native consultancy won’t just sell people. It will sell platforms, models, and intelligent extensions.
A realistic example: Delivery, reimagined
Let’s take a scenario: a mobility client asks a consultancy to design a new digital platform for vehicle servicing.
The traditional model:
- 5–7 people working over 8–12 weeks
- Discovery, research, strategy, design, and tech handled in structured phases, with some overlap
- Progress gated by stakeholder input, multiple rounds of documentation, and alignment checkpoints
- Significant time spent on research collation, synthesis, presentation decks, and translation into user stories and flows
The AI-native alternative
Now imagine the same project—delivered by a lean team of 3–4 people, supported by an AI-powered delivery stack built around orchestrated workflows, not isolated tasks.
Instead of stringing together tools, they run coordinated agent flows that combine research, synthesis, creation, and validation in real time.
Here’s what that looks like in motion:
A research agent powered by Perplexity Pro and custom retrieval pipelines maps the competitive landscape and benchmarks features in hours, not days
A synthesis agent using Claude 3 Opus processes stakeholder transcripts—surfacing sentiment, priorities, contradictions, and unspoken tensions
A content generation agent built on GPT-4-turbo and structured prompt libraries generates job stories, tone-appropriate messaging, and early copy directions
A design support agent orchestrates Diagram and Figma plugins to scaffold editable wireframes based on flows, use cases, and constraints
A documentation agent uses a fine-tuned GPT model to generate user stories in the client’s taxonomy, pre-tagged for import into Jira
A validation layer uses synthetic personas and pre-trained UX heuristics to simulate early feedback—spotting issues before the first round of user testing
What ties this together isn’t just the tools. It’s the system: agents passing data, triggering tasks, and escalating where human judgment is needed. By week three, the client isn’t reviewing strategy slides. They’re reviewing real flows, decisions, and launch options.
The conversation shifts from “What have we learned?” To: “What should we release first?”
This isn’t a prototype sprint. It’s delivery—rearchitected.
By week three, the client sees working flows and design outputs—not just a deck.
The conversation shifts from “What have we learned?” to “What can we release first?”
This isn’t a prototype sprint. It’s real delivery—accelerated.
Where this is heading: The next 2–3 years
Right now, most firms are using GenAI to speed up isolated tasks—drafting copy, summarising notes, scaffolding code.
But we’re entering the next phase.
Expect to see:
- Agent stacks coordinating complex, multi-step delivery flows
- LLMs more deeply integrated into platforms like Jira, GitHub, Notion, and Figma—not just as add-ons, but as collaborators
- Role compression: junior-level tasks handled by tools, mid-level roles augmented, senior roles reoriented toward integration, oversight, and orchestration
- Consultancies building their own delivery IP: reusable agents, dashboards, and decision tools that scale how value is created and shown
This won’t take five years. It’s already underway.
Closing: The opportunity is right in front of us
The consultancies that succeed in this next era won’t be the ones with the most AI experiments or the most polished prototypes.
They’ll be the ones that recognise what AI really offers:
A chance to deliver differently—and to stand apart because of it.
They’ll build delivery models that are lean by design.
They’ll move from outputs to outcomes, from decks to decisions.
They’ll embed intelligence into every layer of how they work—research, design, build, iterate.
They’ll learn faster than their clients expect, and ship faster than their competitors can match.
They won’t just use AI.
They’ll change how value is created—at speed, at scale, and in ways that are hard to copy.
That’s what good will look like.
And the firms willing to build that now?
They won’t just stay relevant.
They’ll build an edge into how they work
Originally published on Linkedin Pulse
- David MitchellChief Growth Officer